Living in a ⿻ World
Living in a ⿻ World
Until lately the best thing that I was able to think in favor of civilization…was that it made possible the artist, the poet, the philosopher, and the man of science. But I think that is not the greatest thing. Now I believe that the greatest thing is a matter that comes directly home to us all. When it is said that we are too much occupied with the means of living to live, I answer that the chief worth of civilization is just that it makes the means of living more complex; that it calls for great and combined intellectual efforts, instead of simple, uncoordinated ones, in order that the crowd may be fed and clothed and houses and moved from place to place. Because more complex and intense intellectual efforts mean a fuller and richer life. They mean more life. Life is an end in itself, and the only question as to whether it is worth living is whether you have enough of it. — Oliver Wendell Holmes, 1900[1]
(A)re…atoms independent elements of reality? No…as quantum theory shows: they are defined by their…interactions with the rest of the world…(Q)uantum physics may just be the realization that this ubiquitous relational structure of reality continues all the way down…Reality is not a collection of things, it’s a network of processes. — Carlo Rovelli, 2022[2]
Technology follows science. If we want to understand ⿻ as a vision of what our world could become, we need to start off by understanding ⿻ as a perspective on how the world already is. The Technocratic and Libertarian perspectives are rooted in a science, namely the monist atomism we described in the previous chapter: the belief that a universal set of laws operating on an fundamental set of atoms is the best way to understand the world.
Technocracy has a long history of being justified by science and rationality. The idea of “scientific management” (a.k.a. Taylorism) that became popular in the early 1900s was justified by making analogies between social systems and simple mathematical models, and logic and reason as ways of thinking about them. High modernism in architecture is similarly inspired by the beauty of geometry.[3] Libertarianism also borrows heavily from physics and other sciences: just like particles “take the path of least action”, and evolution maximizes fitness, economic agents “maximize utility”. Every phenomenon in the world, from human societies to the motion of the stars, can, in the monist atomist view, ultimately be reduced to these laws.
These approaches have achieved great successes. Newtonian mechanics explained a range of phenomena and helped inspire the technologies of the industrial revolution. Darwinism is the foundation of modern biology. Economics has been the most influential of the social sciences on public policy. And the Church-Turing vision of “general computation” helped inspire the idea of general-purpose computers that are so broadly used today.
Yet the last century taught us how much progress is possible if we transcend the limitations of monist atomism. Gödel’s Theorem undermined the unity and completeness of mathematics and a range of non-Euclidean geometries are now critical to science.[4] Symbiosis, ecology, and extended evolutionary synthesis undermined “survival of the fittest” as the central biological paradigm and ushered in the age of environmental science. Neuroscience has been reimagined around networks and emergent capabilities and given birth to modern neural networks. What all these share is a focus on complexity, emergence, multi-level organization and multidirectional causality rather than the application of a universal set of laws to a single type of atomic entity.
⿻ approaches social systems similarly. A corporation plays in the game of global competition, yet is simultaneously itself a game played by employees, shareholders, management and customers. There is no reason to expect the resulting outcomes often to cohere as preferences. What's more, many games intersect: employees of a corporation are often each influenced through their other relationships with the outside world (e.g. political, social, religious, ethnic), and not only through the corporation itself. Countries too are both games and players, intersected by corporations, religions and much more, and there too we cannot cleanly separate apart actions between countries and actions within a country: the writing of this very book is a complex mix of both in multiple ways.
⿻ is thus heavy with analogies to the last century of natural sciences. Drawing out a ⿻ of these influences and analogies, without taking any too literally or universally, allows us to glimpse an inviting path ahead of inspiration and recombination. While Libertarianism and Technocracy can be seen as ideological caricatures, they can also be understood in scientific terms as ever-present threats to complexity.
Essentially every complex system, from the flow of fluids to the development of ecosystems to the functioning of the brain, can exhibit both "chaotic" states (where activity is essentially random) and "orderly" states (where patterns are static and rigid). There is almost always some parameter (such as heat or the mutation rate) that conditions which state arises, with chaos happening for high values and order for low values. When the parameter is very close to the "critical value" of transition between these states, when it sits on what complexity theorists call the "edge of chaos", complex behavior can emerge, forming unpredictable, developing, life-like structures that are neither chaotic nor orderly but instead complex.[5] This corresponds closely to the idea we highlighted above of a "narrow corridor" between centralizing and anti-social, Technocratic and Libertarian threats that we have highlighted above.
As such, ⿻ can take from science the crucial importance of steering towards and widening this narrow corridor, a process complexity scientists call "self-organizing criticality". In doing so, we can draw on the wisdom of many sciences, ensuring we are not unduly captured by any one set of analogies.
Mathematics
Nineteenth century mathematics saw the rise of formalism: being precise and rigorous about the definitions and properties of mathematical structures that we are using, so as to avoid inconsistencies and mistakes. At the beginning of the 20th century, there was a hope that mathematics could be “solved”, perhaps even giving a precise algorithm for determining the truth or falsity of any mathematical claim.[6] 20th century mathematics, on the other hand, was characterized by an explosion of complexity and uncertainty.
- Gödel's Theorem: A number of mathematical results from the early 20th century, most notably Gödel's theorem, showed that there are fundamental and irreducible ways in which key parts of mathematics cannot be fully solved. Similarly, Alonzo Church proved that some mathematical problems were “undecidable” by computational processes.[7] This dashed the dream of reducing all of mathematics to computations on basic axioms.
- Computational complexity: Even when reductionism is feasible in principle/theory, the computation required to predict higher-level phenomena based on their components (its computational complexity) is so large that performing it is unlikely to be practically relevant. In some cases, it is believed that the required computation would consume far more resources than could possibly be recovered through the understanding gained by such a reduction. In many real-world use cases, the situation can often be described as a well-studied computational problem where the “optimal” algorithm takes an amount of time growing exponentially in the problem size and thus rules of thumb are almost always used in practice.
- Sensitivity, chaos, and irreducible uncertainty: Many even relatively simple systems have been shown to exhibit “chaotic” behavior. A system is chaotic if a tiny change in the initial conditions translates into radical shifts in its eventual behavior after an extended time has elapsed. The most famous example is weather systems, where it is often said that a butterfly flapping its wings can make the difference in causing a typhoon half-way across the world weeks later.[8] In the presence of such chaotic effects, attempts at prediction via reduction require unachievable degrees of precision. To make matters worse, there are often hard limits to how much precision is feasible known as "the Uncertainty Principle", as precise instruments often interfere with the systems they measure in ways that can lead to important changes due to the sensitivity mentioned previously.
- Fractals: Many mathematical structures have been shown to have similar patterns at very different scales. A good example of this is the Mandelbrot set, generated by repeatedly squaring then adding the same offset to a complex number. These illustrate why breaking structures down to atomic components may obscure rather than illuminate their inherently multi-scale structure.
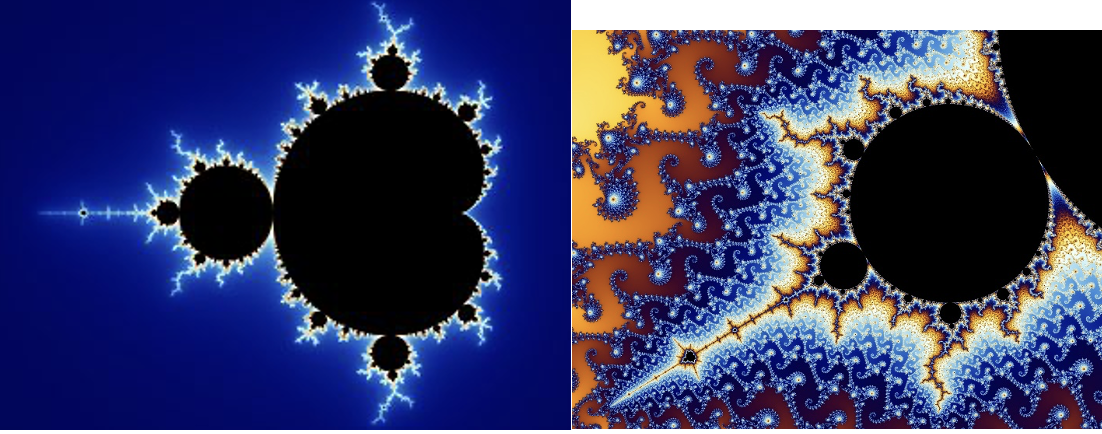
- Relationality in mathematics: in mathematics, different branches are often interconnected, and insights from one area can be applied to another. For instance, algebraic structures are ubiquitous in many branches of mathematics, and they provide a language for expressing and exploring relationships between mathematical objects. The study of algebraic geometry connects these structures to geometry. Moreover, the study of topology is based on understanding the relationships between shapes and their properties. The mix of diversity and interconnectedness is perhaps the defining feature of modern mathematics.
Physics
In 1897, Lord Kelvin infamously proclaimed that “There is nothing new to discover in physics now.” The next century proved, on the contrary, to be the most fertile and revolutionary in the history of the field.
- Einstein's theories of relativity overturned the simplicity of Euclidean geometry and Newtonian dynamics of colliding billiard balls as a guide to understanding the physical world at large scales and fast speeds. When objects travel at large fractions of the speed of light, very different rules start describing their behavior.
- Quantum mechanics and string theory similarly showed that classical physics is insufficient at very small scales. Bell's Theorem demonstrated clearly that quantum physics cannot even be fully described as a consequence of probability theory and hidden information: rather, a particle can be in a combination (or “superposition”) of two states at the same time, where those two states cancel each other out.
- “Heisenberg’s Uncertainty Principle”, mentioned above, puts a firm upper limit on the precision with which the velocity and position of a particle can even be measured.
- The three body problem, now famous after its central role in Liu Cixin's science-fiction series, shows that an interaction of even three bodies, even under simple Newtonian physics, is chaotic enough that its future behavior cannot be predicted with simple mathematical problems. However, we still regularly solve trillion-body problems well enough for everyday use by using seventeenth-century abstractions such as “temperature” and “pressure”.
Perhaps the most striking and consistent feature of the revolutions in twentieth century physics was the way they upset assumptions about a fixed and objective external world. Relativity showed how time, space, acceleration, and even gravity were functions of the relationship among objects, rather than absolute features of an underlying reality. Quantum physics went even further, showing that even these relative relationships are not fixed until observed and thus are fundamentally interactions rather than objects.[9] Thus, modern science often consists of mixing and matching different disciplines to understand different aspects of the physical world at different scales.
The applications of this rich and ⿻ understanding of physical reality are at the very core of the glories and tragedies of the twentieth century. Great powers harnessed the power of the atom to shape world affairs. Global corporations powered unprecedented communications and intelligence by harnessing their understanding of quantum physics to pack ever-tinier electronics into the palms of their customers’ hands. The burning of wood and coal by millions of families has become the cause of ecological devastation, political conflict, and world-spanning social movements based on information derived from microscopic sensors scattered around the world.
Biology
If the defining idea of 19th century macrobiology (concerning advanced organisms and their interactions) was the “natural selection”, the defining idea of the 20th century analog was “ecosystems”. Where natural selection emphasized the “Darwinian” competition for survival in the face of scarce resources, the ecosystem view (closely related to the idea of “extended evolutionary synthesis”) emphasizes:
- Limits to predictability of models: We have continued to discover limits in our ability to make effective models of animal behavior that are based on reductive concepts, such as behaviorism, neuroscience, and so forth, illustrating computational complexity.
- Similarities between organisms and ecosystems: We have discovered that many diverse organisms (“ecosystems”) can exhibit features similar to multicellular life (homeostasis, fragility to destruction or over propagation of internal components, etc.) illustrating emergence and multiscale organization. In fact, many higher level organisms are hard to distinguish from such ecosystems (e.g., multicellular life as cooperation among single-celled organisms or “eusocial” organisms like ants from individual insects). A particular property of the evolution of these organisms is the potential for mutation and selection to occur at all these levels, illustrating multi-scale organization.[10]
- The diversity of cross-species interactions, including traditional competition or predator and prey relationships, but also a range of “mutualism”, where organisms depend on services provided by other organisms and help sustain them in turn, exemplifying entanglement, and relationality.[11]
- Epigenetics: We have discovered that genetics codes only a portion of these behaviors, and “epigenetics” or other environmental features play important roles in evolution and adaptation, showing the multi-level and multidimensional causation inherent even to molecular biology.
This shift was not simply a matter of scientific theory. It led to some of the most important changes in human behavior and interaction with nature of the twentieth century. In particular, the environmental movement and the efforts it created to protect ecosystems, biodiversity, the ozone layer, and the climate all emerged from and have relied heavily on this science of “ecology”, to the point where this movement is often given that label.
Neuroscience
Modern neuroscience started in the late 19th century, when Camillo Golgi, Santiago Ramón y Cajal, and collaborators isolated neurons and their electrical activations as the fundamental functional unit of the brain. This analysis was refined into clear physical models by the work of Alan Hodgkin and Andrew Huxley, who built and tested on animals their electrical theories of nervous communication. More recently, however, we have seen a series of discoveries that put chaos and complexity theory at the core of how the brain functions:
- Distribution of brain functions: mathematical modeling, brain imaging, and single-neuron activation experiments suggested that many if not most brain functions are distributed across regions of the brain, emerging from patterns of interactions rather than primarily physical localization.
- The Hebbian model of connections, where they are strengthened by repeated co-firing, is perhaps one of the most elegant illustrations of the idea of “relationality” in science, closely paralleling the way we typically imagine human relationships developing
- Study of artificial neural networks: As early as the late 1950s, researchers beginning with Frank Rosenblatt built the first “artificial neural network” models of the brain. Neural networks have become the foundation of the recent advances in “artificial intelligence”. Networks of trillions of nodes, each operating on fairly simple principles inspired by neurons of activation triggered by crossing a threshold determined by a linear combination of inputs, are the backbone of the “foundation models” such as BERT and the GPT models.
From science to society
⿻ is, scientifically, the application of an analogous perspective to the understanding of human societies and, technologically, the attempt to build formal information and governance systems that account for and resemble these structures as physical technologies built on ⿻ science do. Perhaps the crispest articulation of this vision appears in the work of the leading figure of network sociology, Mark Granovetter.[12] There is no basic individual atom; personal identity fundamentally arises from social relationships and connections. Nor is there any fixed collective or even set of collectives: social groups do and must constantly shift and reconfigure. This bidirectional equilibrium between the diversity of people and the social groups they create is the essence of ⿻ social science.
Moreover, these social groups exist at a variety of intersecting and non-hierarchical scales. Families, clubs, towns, provinces, religious groups of all sizes, businesses at every scale, demographic identities (gender, sexual identity, race, ethnicity, etc.), education and academic training, and many more co-existing and intersecting. For example, from the perspective of global Catholicism, the US is an important but “minority” country, with only about 6% of all Catholics living in the US; but the same could be said about Catholicism from the perspective of the US, with about 23% of Americans being Catholic.[13]
While we do not have the space to review it in detail, a rich literature provides quantitative and social scientific evidence for the explanatory power of the ⿻ perspective [14]. Studies of industrial dynamics, of social and behavioral psychology, of economic development, of organizational cohesion, and much else, have shown the central role of social relationships that create and harness diversity[15]. Instead, we will pull out just one example that perhaps will be both the most surprising and most related to the scientific themes above: the evolution of scientific knowledge itself.
A growing interdisciplinary academic field of “Metascience” studies the emergence of scientific knowledge as a complex system from networks among scientists and ideas.[16] It charts the emergence and proliferation of scientific fields, sources of scientific novelty and progress, the strategies of exploration scientists choose, and the impact of social structure on intellectual advancement. Among other things, they find that scientific exploration is biased towards topics that have been frequently discussed within a field and constrained by social and institutional connections among scientists, which diminishes the efficiency of the scientific knowledge discovery process.[17] Furthermore, they discover that a decentralized scientific community, made up of mostly independent, non-overlapping teams that use a variety of methods and draw upon a broad spectrum of earlier publications, tends to yield more reliable scientific knowledge. In contrast, centralized communities marked by repeated collaborations and restricted to a limited range of approaches from previous studies are likely to generate less reliable outcomes [18] [19] It also finds strong connections between research team size and hierarchy with the types of findings (risky and revolutionary v. normal science) developed and documents the increasingly dominant role of teams (as opposed to individual research) in modern science.[20] Although the largest innovations tend to arise from a strong grounding in existing disciplines deployed in unusual and surprising combinations [21] [22] [23], it illustrates that most incentive structures used in science (based e.g. on publication quality and citation count) create perverse incentives that limit scientific creativity. These findings have led to the development of new metrics in scientific communities that can reward innovations and offset these biases, creating a more ⿻ incentive set.[24]
Science policy research that directly accounts for and enhances ⿻ in science demonstrates advantages for both the rigor of existing knowledge and the discovery of novel insights. When more distinct communities and their approaches work to validate existing claims, those independent perspectives ensure their findings are more robust to rebuttal and revision. Moreover, when building analytic models based on ⿻ principles by simulating the diversity we see in the most ⿻ scientific ventures, discoveries exceed those produced by normal human science.[25]
Thus, even in understanding the very practice of science, a ⿻ perspective, grounded in many intersecting levels of social organization, is critical. Science of science findings regarding the driving forces behind the emergence of disruptive, innovative knowledge have been replicated in other communities of creative collaboration, such as patents and software projects in GitHub, revealing that a ⿻ outlook could transcend the advance of science and technology of any flavor.
A future ⿻?
Yet the assumptions on which the Technocratic and Libertarian visions of the future discussed above diverge sharply from such ⿻ foundations.
In the Technocratic vision we discussed in the previous chapter, the “messiness” of existing administrative systems is to be replaced by a massive-scale, unified, rational, scientific, artificially intelligent planning system. Transcending locality and social diversity, this unified agent is imagined to give “unbiased” answers to any economic and social problem, transcending social cleavages and differences. As such, it seeks to at best paper over and at worst erase, rather than fostering and harnessing, the social diversity and heterogeneity that ⿻ social science sees as defining the very objects of interest, engagement, and value.
In the Libertarian vision, the sovereignty of the atomistic individual (or in some versions, a homogeneous and tightly aligned group of individuals) is the central aspiration. Social relations are best understood in terms of “customers”, “exit” and other capitalist dynamics. Democracy and other means of coping with diversity are viewed as failure modes for systems that do not achieve sufficient alignment and freedom.
But these cannot be the only paths forward. ⿻ science has shown us the power of harnessing a ⿻ understanding of the world to build physical technology. We have to ask what a society and information technology built on an analogous understanding of human societies would look like. Luckily, the twentieth century saw the systematic development of such a vision, from philosophical and social scientific foundations to the beginnings of technological expression.
Harper’s Magazine. “Holmes – Life as Art,” May 2, 2009. https://harpers.org/2009/05/holmes-life-as-art/. ↩︎
Carlo Rovelli, “The Big Idea: Why Relationships Are the Key to Existence.” The Guardian, September 5, 2022, sec. Books. https://www.theguardian.com/books/2022/sep/05/the-big-idea-why-relationships-are-the-key-to-existence. ↩︎
James C. Scott, Seeing Like a State: How Certain Schemes to Improve the Human Condition Have Failed (New Haven, CT: Yale University Press, 1999). ↩︎
Cris Moore and John Kaag, "The Uncertainty Principle", The American Scholar March 2, 2020 https://theamericanscholar.org/the-uncertainty-principle/. ↩︎
M. Mitchell Waldrop, Complexity: The Emerging Science at the Edge of Order and Chaos (New York: Open Road Media, 2019). ↩︎
Alfred North Whitehead and Bertrand Russell, Principia Mathematica (Cambridge, UK: Cambridge University Press, 1910). ↩︎
Alonzo Church, "A note on the Entscheidungsproblem", The Journal of Symbolic Logic 1, no. 1: 40-41. ↩︎
James Gleick, Chaos: Making a New Science (New York: Penguin, 2018). ↩︎
Carlo Rovelli, "Relational Quantum Mechanics", International Journal of Theoretical Physics 35, 1996: 1637-1678. ↩︎
David Sloan Wilson and Edward O. Wilson, "Rethinking the Theoretical Foundation of Sociobiology" Quarterly Review of Biology 82, no. 4, 2007: 327-348. ↩︎
These discoveries have continually and deeply intertwined with ⿻ social thought, from "mutualism" being used almost interchangeably by early anarchist thinkers like Pierre-Joseph Proudhon to one of the authors of this book publishing his second paper on biological mutualism, then developing these ideas further into the theories we will return to in our chapter on Social Markets. Pierre-Joseph Proudhon, System of Economic Contradictions (1846). E. Glen Weyl,Megan E. Frederickson, Douglas W. Yu and Naomi E. Pierce, "Economic Contract Theory Tests Models of Mutualism" Proceedings of the National Academy of Sciences 107, no. 36, 2010: 15712-15716. ↩︎
Mark Granovetter, "Economic Action and Social Structure: The Problem of Embeddedness", American Journal of Sociology 91, no. 3 (1985): 481-510. ↩︎
Pew Research Center, "The Global Catholic Population", February 13, 2013 https://www.pewresearch.org/religion/2013/02/13/the-global-catholic-population/. ↩︎
In assemblage theory, as articulated by Manuel DeLanda, entities are understood as complex structures formed from the symbiotic relationship between heterogeneous components, rather than being reducible to their individual parts. Its central thesis is that people do not act exclusively by themselves, and instead human action requires complex socio-material interdependencies. DeLanda's perspective shifts the focus from inherent qualities of entities to the dynamic processes and interactions that give rise to emergent properties within networks of relations. His book "A New Philosophy of Society: Assemblage Theory and Social Complexity" (2006) is a good starting point. ↩︎
Scott Page, The Difference: How the Power of Diversity Creates Better Groups, Firms, Schools, and Societies, (Princeton: Princeton University Press, 2007); César Hidalgo, Why Information Grows: The Evolution of Order, from Atoms to Economies, (New York: Basic Books, 2015); Daron Acemoglu, and Joshua Linn, “Market Size in Innovation: Theory and Evidence from the Pharmaceutical Industry,” Library Union Catalog of Bavaria, (Berlin and Brandenburg: B3Kat Repository, October 1, 2003), https://doi.org/10.3386/w10038; Mark Granovetter, “The Strength of Weak Ties,” American Journal of Sociology 78, no. 6 (May 1973): 1360–80; Brian Uzzi, “Social Structure and Competition in Interfirm Networks: The Paradox of Embeddedness,” Administrative Science Quarterly 42, no. 1 (March 1997): 35–67. https://doi.org/10.2307/2393808; Jonathan Michie, and Ronald S. Burt, “Structural Holes: The Social Structure of Competition,” The Economic Journal 104, no. 424 (May 1994): 685. https://doi.org/10.2307/2234645; McPherson, Miller, Lynn Smith-Lovin, and James M Cook. “Birds of a Feather: Homophily in Social Networks.” Annual Review of Sociology 27, no. 1 (August 2001): 415–44. ↩︎
Santo Fortunato, Carl T. Bergstrom, Katy Borner, James A. Evans, Dirk Helbing, Stasa Milojevič, Filippo Radicchi, Robeta Sinatra, Brian Uzzi, Alessandro Vespignani, Ludo Waltman, Dashun Wang and Alberto-László Barbási, "Science of Science" Nature 359, no. 6379 (2018): eaao0185. ↩︎
Andrey Rzhetsky, Jacob Foster, Ian Foster, and James Evans, “Choosing Experiments to Accelerate Collective Discovery,” Proceedings of the National Academy of Sciences 112, no. 47 (November 9, 2015): 14569–74. https://doi.org/10.1073/pnas.1509757112. ↩︎
Valentin Danchev, Andrey Rzhetsky, and James A Evans, “Centralized Scientific Communities Are Less Likely to Generate Replicable Results.” ELife 8 (July 2, 2019), https://doi.org/10.7554/elife.43094. ↩︎
Alexander Belikov, Andrey Rzhetsky, and James Evans, "Prediction of robust scientific facts from literature," Nature Machine Intelligence 4.5 (2022): 445-454. ↩︎
Lingfei Wu, Dashun Wang, and James Evans, "Large teams develop and small teams disrupt science and technology," Nature 566.7744 (2019): 378-382. ↩︎
Yiling Lin, James Evans, and Lingfei Wu, "New directions in science emerge from disconnection and discord," Journal of Informetrics 16.1 (2022): 101234. ↩︎
Feng Shi, and James Evans, "Surprising combinations of research contents and contexts are related to impact and emerge with scientific outsiders from distant disciplines," Nature Communications 14.1 (2023): 1641. ↩︎
Jacob Foster, Andrey Rzhetsky, and James A. Evans, "Tradition and Innovation in Scientists’ Research Strategies," American Sociological Review 80.5 (2015): 875-908. ↩︎
Aaron Clauset, Daniel Larremore, and Roberta Sinatra, "Data-driven predictions in the science of science," Science 355.6324 (2017): 477-480. ↩︎
Jamshid Sourati, and James Evans, "Accelerating science with human-aware artificial intelligence," Nature Human Behaviour 7.10 (2023): 1682-1696. ↩︎